COCO IS "ALL" YOU NEED FOR VISUAL INSTRUCTION FINE-TUNING
COCO IS "ALL" YOU NEED FOR VISUAL INSTRUCTION FINE-TUNING
Introduction
In this work, we establish a new IFT dataset, with images sourced from the COCO dataset along with more diverse instructions. Our experiments show that when fine-tuned with out proposed dataset, MLLMs achieve better performance on open-ended evaluation benchmarks in both single-round and multi-round dialog setting.
Motivation
- Multi-round dialogs are expensive to construct.
- Current instruction following data makes the model overfit to single instruction.
- Multi-round evaluation benchmark is essential for evaluating the quality of instruction following.
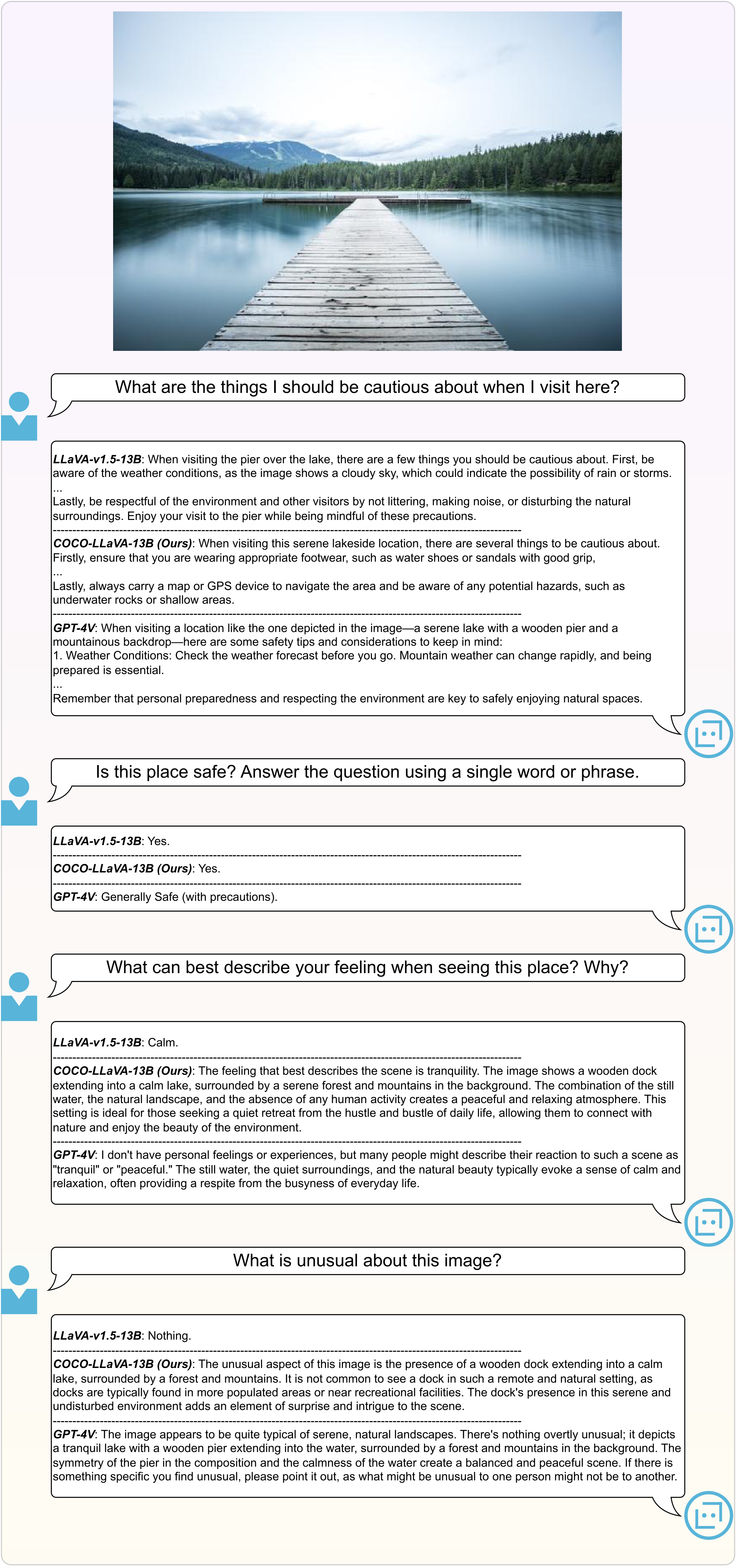
Dataset summarization
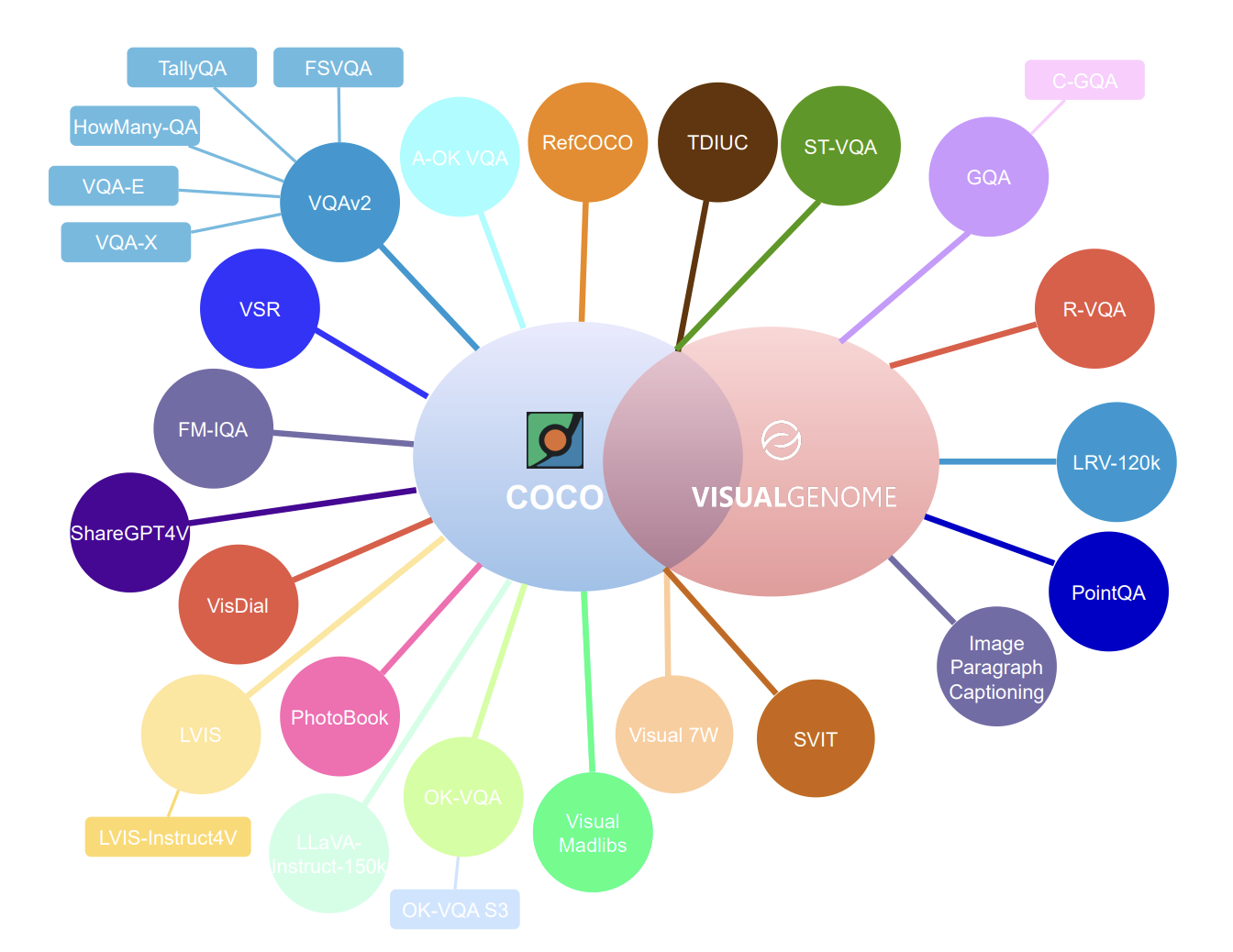
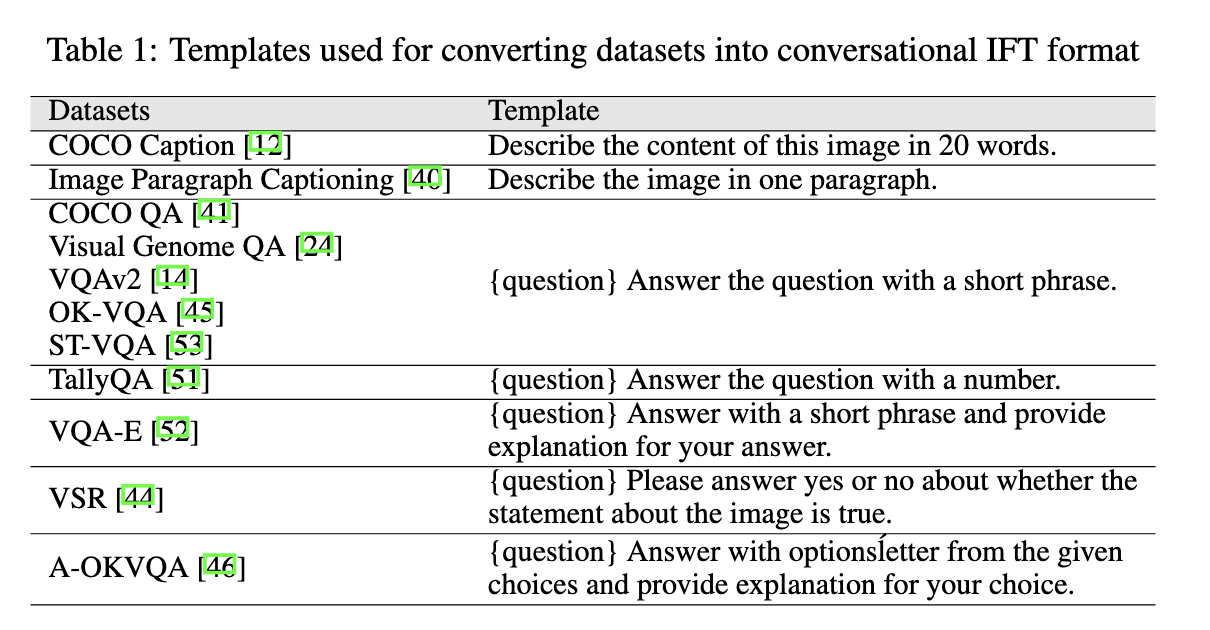
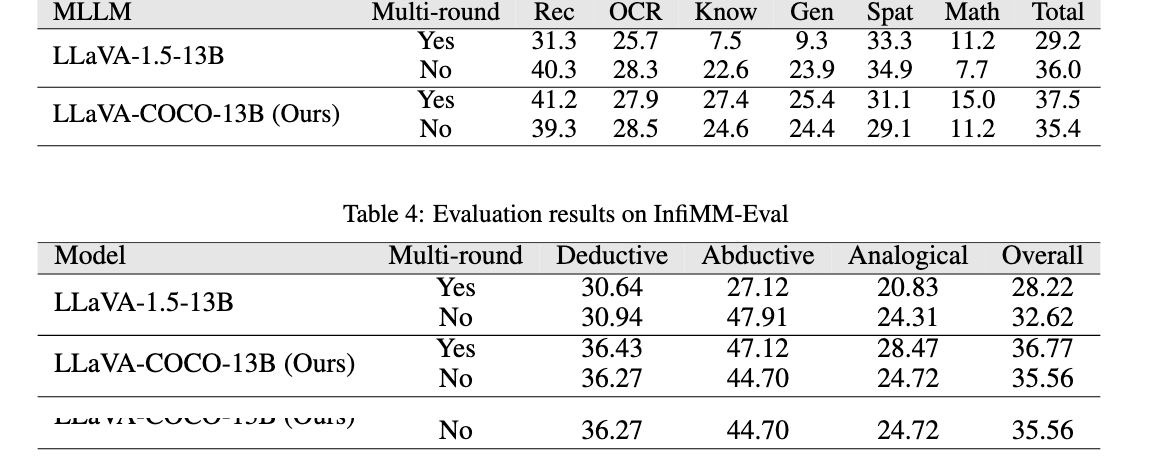
Conclusions
This overfitting leads to a degradation in performance in multi-round dialog settings. We construct an IFT dataset by simply merging datasets with COCO images. Experiments show that models trained with our dataset demonstrate better instruction-following ability and achieve equal or better performance on open-ended evaluation benchmarks. The results suggest that the COCO dataset is ``all’’ you need for visual IFT. We call for more comprehensive research to better understand IFT dataset construction, better evaluation benchmarks for modern open-ended MLLMs rather than traditional caption and VQA benchmarks.